How Machine Learning Drives Artificial Lift Performance
Unplanned downtime in the oil and gas industry leads to costly problems like production delays. With artificial lift downtime being a primary source of such deferred production, identifying potential problems before they occur is crucial.
By applying advanced analytics and machine learning (ML) at scale in the cloud, oil and gas companies can improve their field performance. Using asset diagnostics and acting on real-time monitoring insights, you’ll create data-driven maintenance flows, leading to an increase in production, reduced carbon footprint, and reduced lease operating expenses.
This digital event will explain how AWS production monitoring solutions, and services like Amazon SageMaker reduce unscheduled maintenance and deferred production. These tools help you to predict suboptimal equipment performance and potential failures so you can make data-driven operational decisions.
Why watch? Hear from Mohamed Shawky, Business Development Principal for Energy at AWS, and David Benham, Senior Data Scientist at Laredo Petroleum, as they discuss the challenges of rotating equipment performance in the context of artificial lift. These energy experts will then share how using telemetry data analysis and machine learning modeling helps solve these issues.
You’ll also hear from Yaroslav Svyryda, a data scientist, and Andrii Struk, Energy, Oil, and Gas SMEs at SoftServe. They will demonstrate the Proof-of-Concept (PoC) created in collaboration with Laredo Petroleum and AWS. The POC’s purpose was to optimize Laredo’s artificial lift compressors performance as well as predict any anomalies or failures. Learn how this POC utilized exploratory data analysis (EDA), data processing, and ML modeling using Amazon SageMaker, ultimately increasing Laredo’s equipment uptime and reliability.
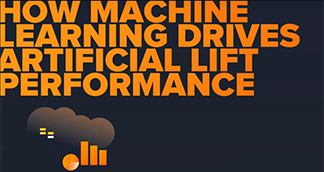